Enterprise AI Adoption Update
Last week saw the publication of an interesting research report from the Asana Work Innovation Lab and Anthropic, which surveyed 5000+ individuals in the UK and USA to assess the current state of AI adoption in the enterprise. The report found that adoption has surged by 44% in nine months, with more than half of knowledge workers now using generative AI in some way, and self-reported productivity improvements were twice as likely for daily users (89%) compared to those who only dabble once a month or so (39%). Definitely worth a read (and also nicely designed).
But the report also goes on talk about significant adoption challenges, such as
- AI literacy is frighteningly low, with 64% of workers having little to no familiarity with AI tools
- Only 31% of companies have a formal AI strategy in place
- Dangerous divides exist between executives and individual contributors in terms of AI enthusiasm, adoption, and perceived benefits
It classified adoption using five levels, from skepticism through to maturity, and found that organisations with a high degree of AI maturity tended to do the following:
- Invest in learning and AI literacy
- Share role-based use case libraries
- Identify ‘champions’ and make it easy to access subject experts
- Create sandboxes and safe zones for experimentation
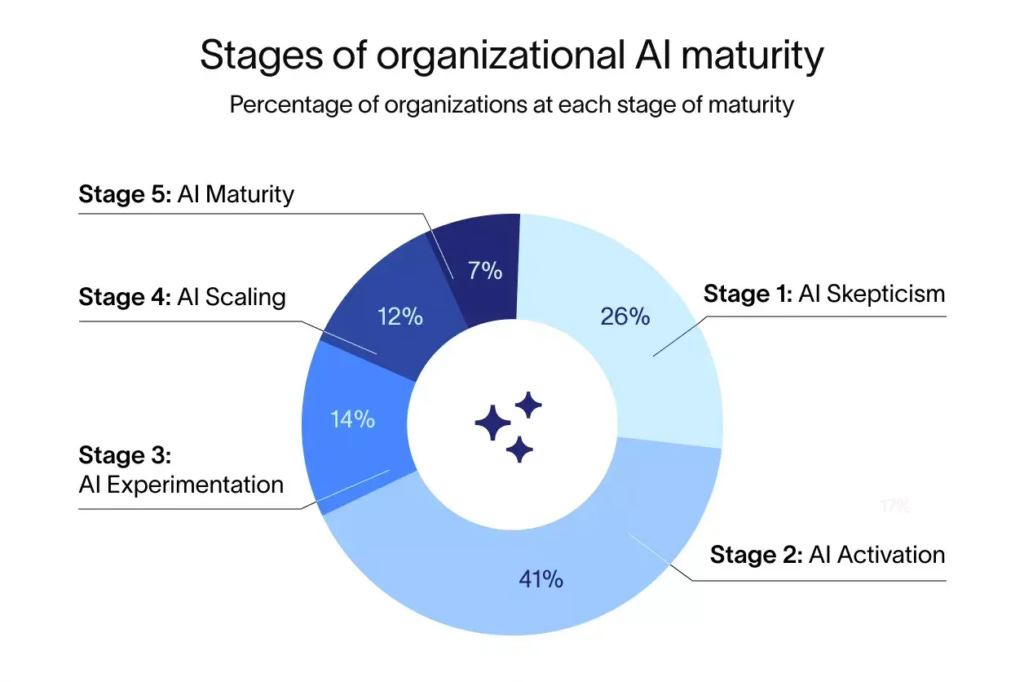
From ‘The State of AI at Work 2024’ by Rebecca Hinds
From Simple Use Cases to Multi-Agent Systems
This is all sensible stuff, but it is worth qualifying the broadly positive findings of the report by pointing out that this is mostly about individual uses of generative AI for simple tasks, such as content generation and summarisation, rather than complex process automation or the creation of new AI apps and AI-enhanced services.
Anecdotally, using GenAI for coding seems to produce even higher productivity improvements, so it will be fascinating to see which organisations are able to extend this to no-code prototyping outside of tech functions, as we wrote about previously.
But the next stage of adoption is probably about agent-based AI tools and helpers that are always running in the background to help people find and process information, connect with others, or perform multi-stage process tasks. And this will not be about finding the one best LLM or system, but more likely a multi-agent approach where specific agents hand-off to each other based on their specialisation and training.
I am enjoying Joanne Chen’s exploration of this area over at Foundation Capital. She recently interviewed two AI researchers working in this domain, and both conversations are quite educational in terms of the potential for multi-agent systems to transform the way work is done inside organisations:
- How to Build Generative AI Agents, with Joon Sung Park at Stanford (video here)
- The Promise of Multi-Agent AI, with Chi Wang of Microsoft (video here)
Apple Enters the Chat
This week’s big AI news was Apple’s announcement of its own AI (Apple Intelligence) at its WWDC event. There will be a lot of speculation about how this will play out, especially the link with OpenAI, but I think this could be an important development for reasons that go way beyond the battle between individual LLMs:
- The focus on user experience, rather than LLM parameter size, will give Apple an opportunity to demonstrate the power of AI agents at scale using a secure, on-device small language model.
- The focus on security and privacy, with an end-to-end pathway from device to private cloud all on Apple silicon, plus optional calls to OpenAI (or other LLMs), where users are comfortable with that, could help prove the case for personal AI agents.
- Having all of a user’s most personal information available for (secure, on-device) training allows for highly contextual concierge and personal productivity features, especially for iCloud customers who use docs, music, activity, news and other services in addition to calendars, messages, etc., whilst reducing the scope for hallucinations.
- The approach of sprinkling AI adapters and integrations across the various apps on the iOS platform, rather than just trying to make Siri a single all-powerful AI app, makes great use of their platform and could help showcase the benefits of narrow AI agents with specific use cases. AI as a feature not a product. Lots of little helpers that augment and elevate what we want to do.
As a caveat, it seems Apple is taking a slow and cautious second- or third-mover approach to AI, so this might take time. But demonstrating the art of the possible for consumers in their ecosystem could become an example of what is possible in a work context as well, whilst demonstrating that LLM size matters less than use cases, experience and the capabilities we wrap around them.
Paradigm Shifts When?
This all makes enterprise AI adoption feel even more like the poor relation to the consumer world, which is frustrating given the immense value waiting to be unlocked in that domain.
Imagine if your organisation was like the iOS platform, with all its underlying core functions and services integrated within a secure protected environment with a connected data platform, and teams could operate freely on top – as apps do in iOS – using these services to innovate, connect and get work done. Now imagine you could do what Apple seems to be doing and sprinkle narrow, contextual AI augmentation across these teams and services to further enhance and augment the work they do, and gradually automate the basics.
The key reason previous waves of technology-led organisational innovation have not realised their promise is because they have been retro-fitted to the old management and organisational architecture. This is one of the biggest risks for enterprise adoption trying to make our dumb process work faster and more efficiently, rather than automating and integrating the boring stuff so that people are more free to focus on innovating and building value on top.
A recent example of this is the press coverage generated last week by Zoom’s CEO predicting avatars will soon attend meetings on our behalf. Sorry to state the obvious, but I think the goal is not to have those kind of meetings at all, and of course AIs don’t need to put on a shirt, pull up a chair and make small talk about the weather, football or Love Island before they ‘go round the room’ and finally exchange some information. Such basic exchanges will take 45ms not 45 minutes – and will not require biscuits.
Just as the resistance from old school managers to the remote / online working trend is indicative of a deeper malaise in their culture and practice, we can probably also expect many more lazy attempts to shoehorn AI adoption into the control structures and ‘manual’ ways of working that such managers are wedded to as the only way to run their functions.
LearnOps & ChangeOps, not just DevOps & MLOps
The potential for enterprise AI to reduce costs and accelerate (even automate) value creation is huge, whilst also humanising organisations and improving employee experience by allowing people to focus on what they do best, rather than turning them into process work drones.
But it will involve an unbundling and re-bundling of roles, tasks and jobs to be done, and a re-imagining of many roles we take for granted today, including management. It will also require a re-architecting of control and coordination systems from manual command and control systems to connected, extensible platform structures that make available the services, agents and data people need to get things done. A retro-fit won’t cut it this time around.
When we sit down and design the new business capabilities that LLMs, agents, automations and smart services make possible, we need to think a lot more broadly than just the software. We need to think about the platform and how to govern, integrate and connect the services and capabilities we create. And most of all we need to think about people. What learning do we need to wrap around new capabilities to support the transition for employees, and how do we guide and support the change process?
Likely, we will also need to re-define our approaches to learning and change so that they are no longer an add-on or an afterthought. Just as we saw with maintainability and scaling in DevOps, we need to build them in from the very beginning. But we will also need these work streams to be a lot more agile and adaptive than they have been in the past, probably also supported by better tech and experience design.
This is something we are thinking about a lot. What would a no-code development environment for new capabilities look like, and how could it be simple enough for any team to operate, whilst giving them an integrated view of the service design, learning, change and continuous improvement needed to make these new capabilities the building block of an organisation for the age of algorithms?
Apple, of course, has some brilliant designers steeped in a long culture of outside-in user focus, and they are good at imagining the simple apps and experiences that bring technology to life. But on a simpler, more mundane level, we also need this kind of design and development approach for organisational functions and capabilities if we are to get the best out of our magical emerging technologies.