There was another important open model release this week, Alibaba’s QWEN 3, which seems to be an impressive release that relies on strong reasoning capabilities to bring up its benchmark scores. As Nathan Lambert explains, the variety of post-training methods being applied to base models these days can make it hard to evaluate them side-by-side:
The differentiating factor right now across models is in the post-training, especially with reasoning enabling inference-time compute that can take an evaluation score from a 40% range to an 80% range. What denotes a “base model” above is also very messy, as a lot of mid-training is done on these models, where they see instruction and reasoning data in order to prepare for post-training.
He goes on to point out how the rapid rise of open weight models from China might have geo-political implications related to the trade war launched by the US President.
In many ways, open-weight AI models seem like the most effective way for Chinese companies to gain market share in the United States. These models are just huge lists of numbers and will never be able to send data to China, but they still convey extreme business value without opening the rabbit holes of concern over sending any data to a Chinese company….
These open-weight Chinese companies are doing a fantastic job of exerting soft power on the American AI ecosystem. We can all benefit from them technologically.
In the context of Washington’s attempts to crack down on DeepSeek, and also its chip suppier NVIDIA, this suggests restrictions might backfire on the US Government and could perversely accelerate Chinese innovation by incentivising less compute-intensive techniques. Ironically, NVIDIA has also just launched a model based on the QWEN base that is optimised for mathematical reasoning, which shows how innovation ultimately doesn’t care for nationalism and borders.
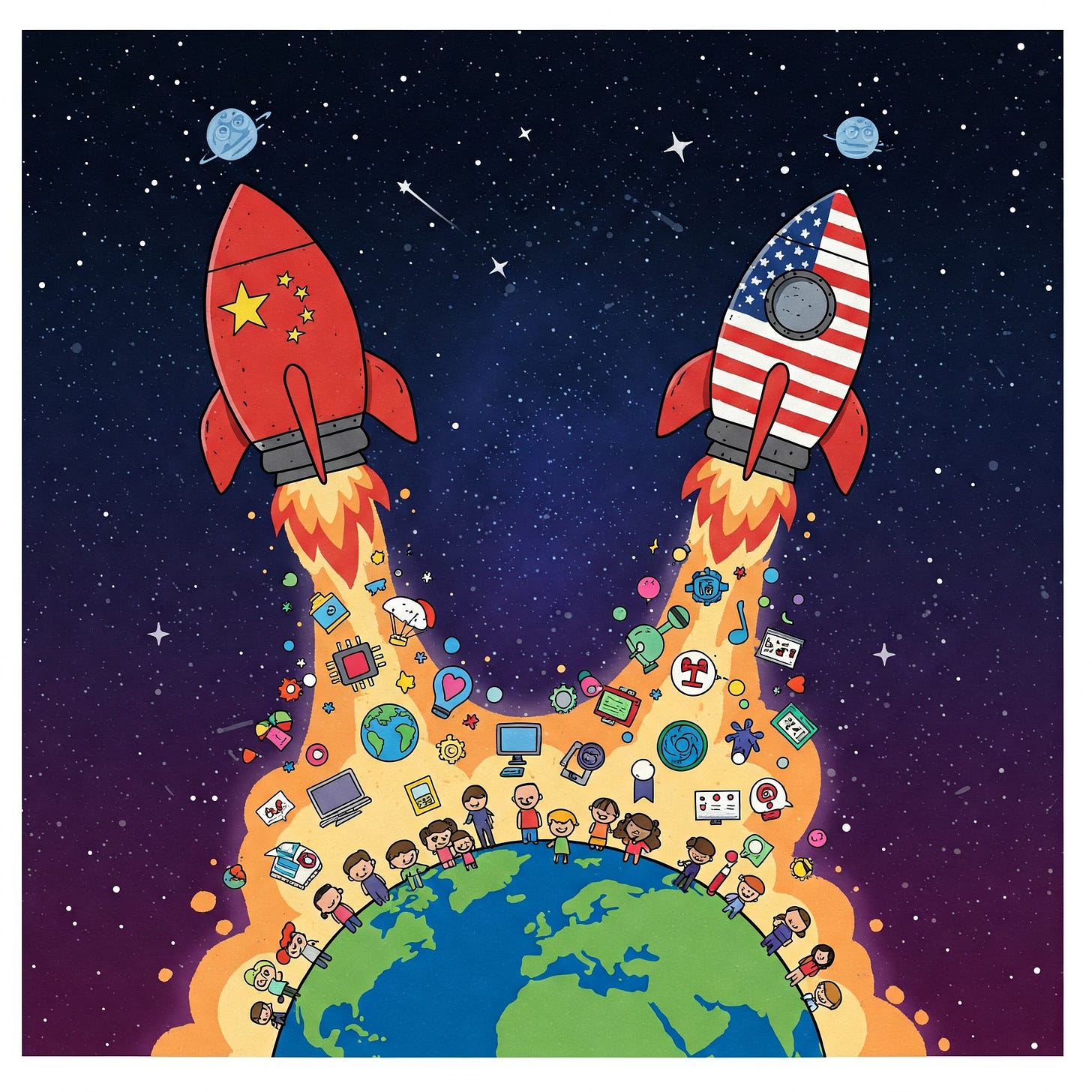
A New Space Race?
In response to US attempts to hold back China’s AI development, President Xi Jinping has called for Chinese self-reliance and the reducing their dependency on US technology. It looks like we have a new space race on our hands, but with China having the technological skills and innovative potential to at least match the abundant investment and access to hardware of their adversaries.
A space race sounds great for competitive innovation, and hopefully it will produce inventions that advance all of us. But even with the simplest of space race technologies, there was a time lag between invention and widespread adoption. I asked an LLM to generate a timeline for some of the better known space race inventions, and especially for the main industrial uses, we are talking about a lag of three decades before they became mainstream. This mirrors the example of the earlier electrification of factories, where adoption took four decades from invention to adoption and diffusion.
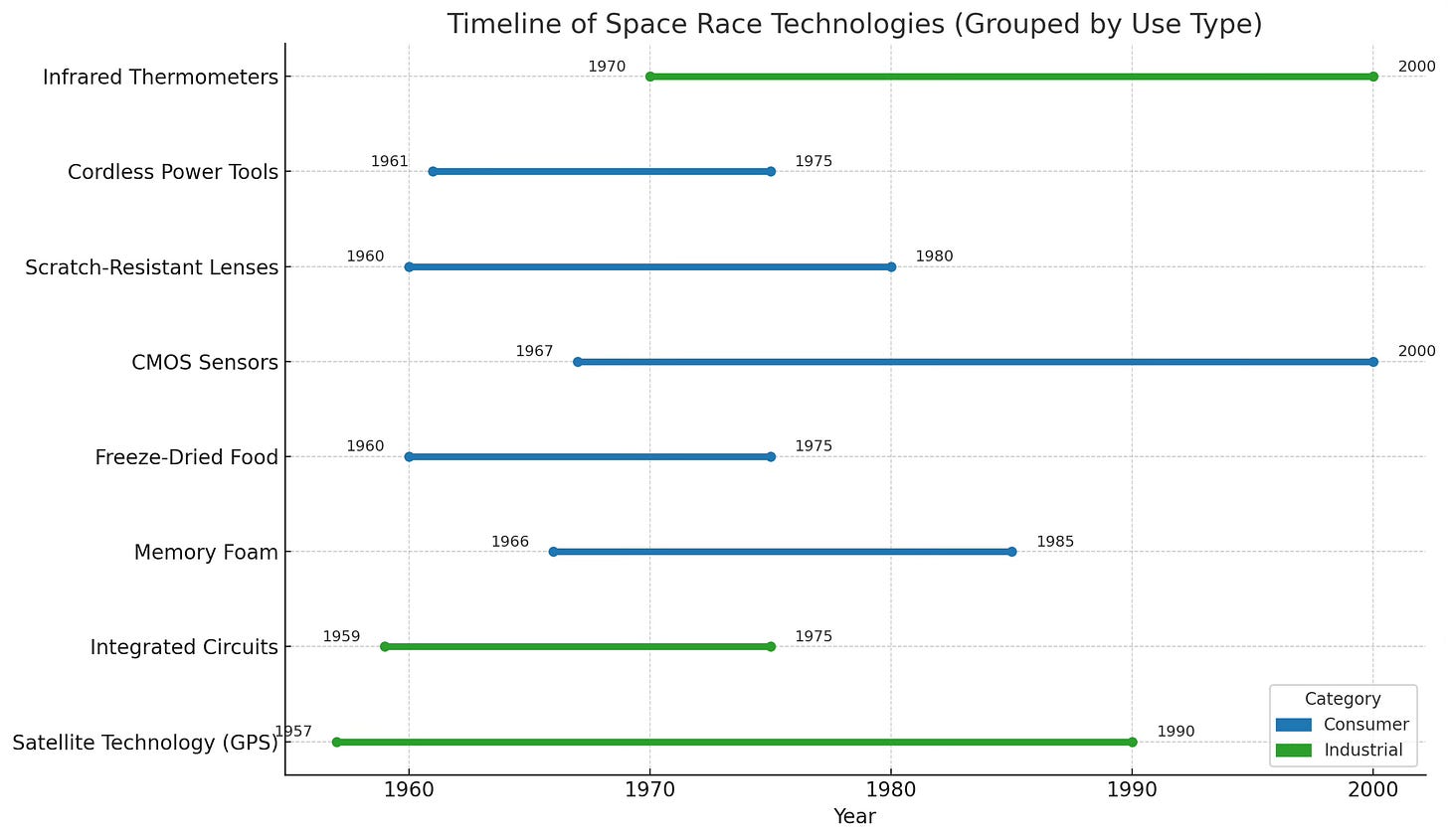
Diffusion & Adoption Work on Human Timescales
Critical voices such as Ed Zitron look at OpenAI’s accounts and conclude they make no sense and the company is doomed; or they look at Microsoft’s acquihire of Mustafa Suleyman, which has not yet demonstrated a breakthrough in adoption of Co-pilot. But the development of base models and the adoption of apps and tools built on them are two distinct phenomena, so we should not be too impatient.
In OpenAI’s case, they are spending spectacular amounts of money to maintain a leading edge position in consumer AI, but whether they ultimately succeed or fail as a business is immaterial. What matters is the technological affordances they have generated; but their adoption is down to us. There are always first movers in a wave of technology-led change who spend heavily to create the infrastructure that later fast followers will ultimately benefit from.
In Microsoft’s case, enterprise AI remains their biggest opportunity – see their recent report on AI-enabled Frontier Firms– especially with so many captive customers reliant on their traditional tech stack. This will take time to come to fruition, and the biggest barrier to adoption will be customer readiness for AI, not the tech itself. Even last gen models and, increasingly, Small Language Models (SLMs) are more than good enough for most purposes.
Paul Sweeney recently criticised the gold rush messaging of consulting firms who argue you must jump on the enterprise AI train or risk being left behind, sharing a list of cautionary tales of failed AI adoption (mostly B2C chatbot fails, which are common). The Wall Street Journal also recently covered Johnson & Johnson’s decision to cut off many of their AI pilots to focus on use cases that were proving useful. But overall, the adage that we tend to over-estimate short-term impact and under-estimate long-term impact probably holds true.
AI as a Normal Technology
Perhaps, as Arvind Narayanan and Sayash Kapoor wrote recently, AI is a normal technology after all, at least in terms of the apps, agents, tools and systems that we build on the magical base models. Sadly, that means we need to do the hard work of readiness, change management, security and so on.
The claim that the speed of technology adoption is not necessarily increasing may seem surprising (or even obviously wrong) given that digital technology can reach billions of devices at once. But it is important to remember that adoption is about software use, not availability. Even if a new AI-based product is instantly released online for anyone to use for free, it takes time to for people to change their workflows and habits to take advantage of the benefits of the new product and to learn to avoid the risks.
Thus, the speed of diffusion is inherently limited by the speed at which not only individuals, but also organizations and institutions, can adapt to technology. This is a trend that we have also seen for past general-purpose technologies: Diffusion occurs over decades, not years.
Ethan Mollick writes about the jagged frontier of AI/AGI, and how models can appear to be geniuses with certain tasks, but idiots with others. The same is true for the adoption of AI, especially in the enterprise. Some will do the hard work of ensuring readiness, identifying and developing early use cases to achieve an advantage, whilst others will struggle adopting new tech into old ways of working.
Perhaps we will need multiple models to overcome the jagged inconsistencies and outright hallucinations that still pop up in certain cases, or perhaps in application areas where we need certainty, techniques like neurosymbolic AI might be enough to solve the problem.
Better still, we might use ecosystems of agents with a degree of competition between them to evolve in the direction of reliable, testable outcomes.
I enjoyed this recent article by Artificiality on how agentic AI can take us on a journey from a simplistic view of intelligence towards one where it is an emergent quality of connected systems and networks, and “societies of agents” with different roles can compete and cooperate to achieve a higher level goal.
This evolution from isolated models to integrated systems points toward increasingly social forms of artificial intelligence, with emerging research revealing intriguing parallels to biological and social systems. Recent experiments demonstrate AI agents spontaneously developing communication protocols, engaging in basic forms of coordination, and even exhibiting rudimentary social behaviors like turn-taking and information sharing. These interactions emerge from the fundamental requirements of solving complex tasks in shared environments.
But to make this work, we have to work on readiness. We need to take a service-centric view of business processes so that we can automate workflows and turn them into digital services that can be invoked by agents. We need to create an architecture of collaboration between agents of different types. And we need to focus on creating and connecting knowledge graphs that represent what an organisation ‘knows’ and how it sees the world, as this recent piece from Diginomica describes. Agents need a map of the world they inhabit, and right now the divided and disconnected nature of corporate knowledge stocks is a barrier to achieving that.