With successive waves of technology innovation and adoption, the initial applications tend to be those that make our current ways of working slightly better, and we measure their success in terms of marginal cost reduction or productivity improvement.
Sometimes, like the internet, a technology is so disruptive that it will go on to change the art of the possible, resulting in a shake-up of business models and entire industries. Sometimes, like the introduction of personal computing in the enterprise, adoption is so closely wrapped up in existing ways of working that the opportunity for re-thinking work based on the new affordances made possible by the technology (e.g. autonomy or decentralisation) is missed – and organisations remain unchanged.
How we choose to adopt and deploy AI in the enterprise today will determine how quickly we get from faster horses to transformative business improvement.
But it is not just organisations that AI looks set to disrupt, but also the market for the enterprise tools and software that help run them. The dominant software business model – Software as a Service – could be challenged as it becomes easier to write and run code, and rather than buy generic software platforms, perhaps companies might build more of their own AI tooling, and buy in (or rent) specialised AI services to augment them.
Rather than software as a service, could service as a software become a viable delivery model for specialist AI? Certainly that is where some leading investors think the market is headed.
AI to shake up Enterprise Software
Sam Lessin of Slow Ventures last week joined a growing chorus of people predicting the end of SaaS, and suggested using software as leverage rather than it being ‘the product’:
If you can build software today that allows you to operate basically any real world business’ 20%-30% better than it is operated today, use that leverage and take over those businesses… take the wins, get the growth, use the margin expansion to dominate your competitors in the actual business.
The most at-risk categories of SaaS are likely to be over-priced systems of record that have come to dominate in areas such as HR, Sales/CRM and ERP.
Systems-of-record are the horse carriages in the age of the automobile – designed for a different job to be done, outmoded, outdated.
Selling service-as-a-software enables a startup to change the way a customer works. Behavior change is hard. But successfully executed, it’s a moat.
Investors in enterprise software and services foresee a shift from selling a one-size fits all generic platform that is ‘good enough’ (usually on a per-seat license) to providing specific service outcomes using software and AI (perhaps on a pay-by-results basis).
Foundation Capital see this as a $4.6tn opportunity:
AI companies are leading a transition from Software-as-a-Service to Service-as-Software, turning the table on the very essence of SaaS. In the software business, a company may sell access to its platform or tool, but customers are still responsible for using that tool to achieve the desired outcome. In the services business, responsibility for achieving the desired outcome sits with the company selling the service. Instead of QuickBooks, you offer tax services—in this case, conducted by an AI accountant. The upside of this change is huge—a $4.6 trillion opportunity, since the global services market dwarfs the software market in size.
But given that many banks and insurance companies are still running mainframes and COBOL, we cannot expect the enterprise software estate of large companies to change overnight. However, AI could play a key role in this transition – even at an early stage – by helping to knit together disparate systems and overcome silos, according to this excellent analysis in Silicon Angle of how agentic AI platforms will redefine enterprise applications:
Agents will work in a coalition with other agents and respond to actions and changes in the market while optimizing for a top-level business goal such as customer satisfaction, market share, profitability or growth. These agents will complement and augment existing automated processes. Importantly, they’ll learn from these existing systems and human actions and do things that can’t effectively be hand-coded.
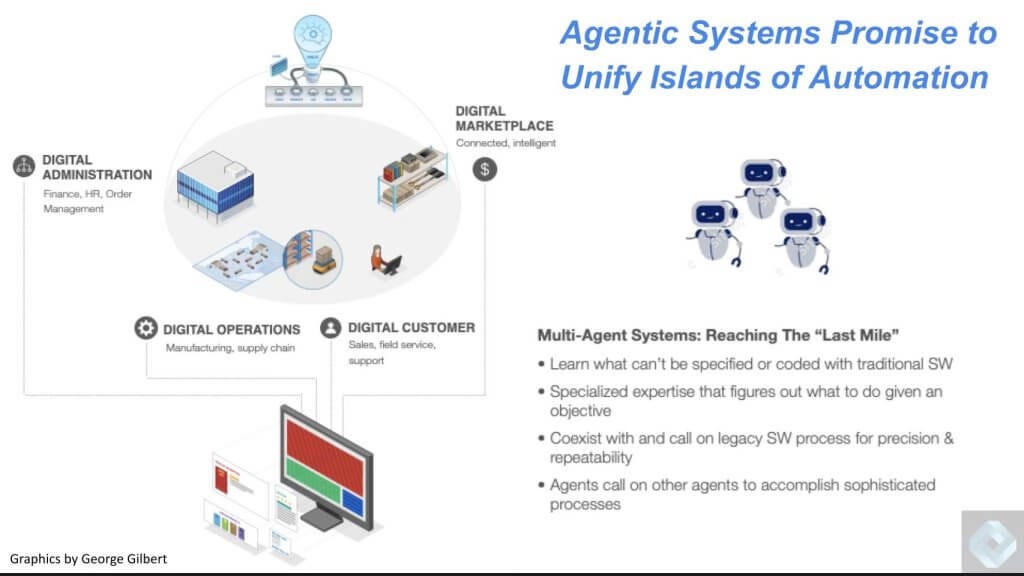
Image from Silicon Angle report linked above
Co-pilots help people; do agents replace them?
Salesforce are among those SaaS incumbents who are trying to embrace this shift by investing heavily in its agent layer that will sit on top of their platform, and also by experimenting with outcome-based charging, rather than per-seat licensing.
But one factor that could delay progress is the readiness of client organisations in both technology/data and also skills/ways of working. If an organisation has not yet integrated or connected its data, that will limit the ability of AI to do clever things with it. And if they are slow or reluctant to embrace new skills and new ways of working, then faster horses and marginal productivity gains might be all they can achieve.
In a fascinating article about the different philosophies underpinning tech firms, Ben Thompson cites the example of Palantir, who realised their clients’ data readiness was a barrier to achieving their mission, and adjusted their approach based on this insight, according to their CTO Shyam Sankar:
I think the critical part of it was really realizing that we had built the original product presupposing that our customers had data integrated, that we could focus on the analytics that came subsequent to having your data integrated. I feel like that founding trauma was realizing that actually everyone claims that their data is integrated, but it is a complete mess and that actually the much more interesting and valuable part of our business was developing technologies that allowed us to productize data integration, instead of having it be like a five-year never ending consulting project, so that we could do the thing we actually started our business to do.
In the piece, Thompson also points to the different philosophies of AI co-pilots and agents – the former exist to help people get their work done, whilst the latter seek to replace human work with automation. But while both require changes to the organisation to make the most of them, co-pilots suffer from a very human behaviour change problem:
… the real challenge for Copilot is that it is a change management problem: it’s one thing to charge $30/month on a per-seat basis to make an amazing new way to work available to all of your employees; it’s another thing entirely — a much more difficult thing — to get all of your employees to change the way they work in order to benefit from your investment, and to make Copilot Pages the “new artifact for the AI age”, in line with the spreadsheet in the personal computer age.
Those workers curious or motivated enough to see what spreadsheets could do in the 1980s, use the web in the 1990s, or adopt social computing in the 2000s will undoubtedly already be playing with AI tools and co-pilots today. But they are in the minority. Perhaps we will see the co-pilot philosophy work well with ‘talent’, enabling smaller teams to produce outsized outcomes, whilst agents quietly hollow out many internal process-following roles, including in management.
But ultimately, Thompson argues that we will need top-down leadership commitment to embracing the possibilities and implications of AI for the enterprise, rather than just organic adoption and usage.
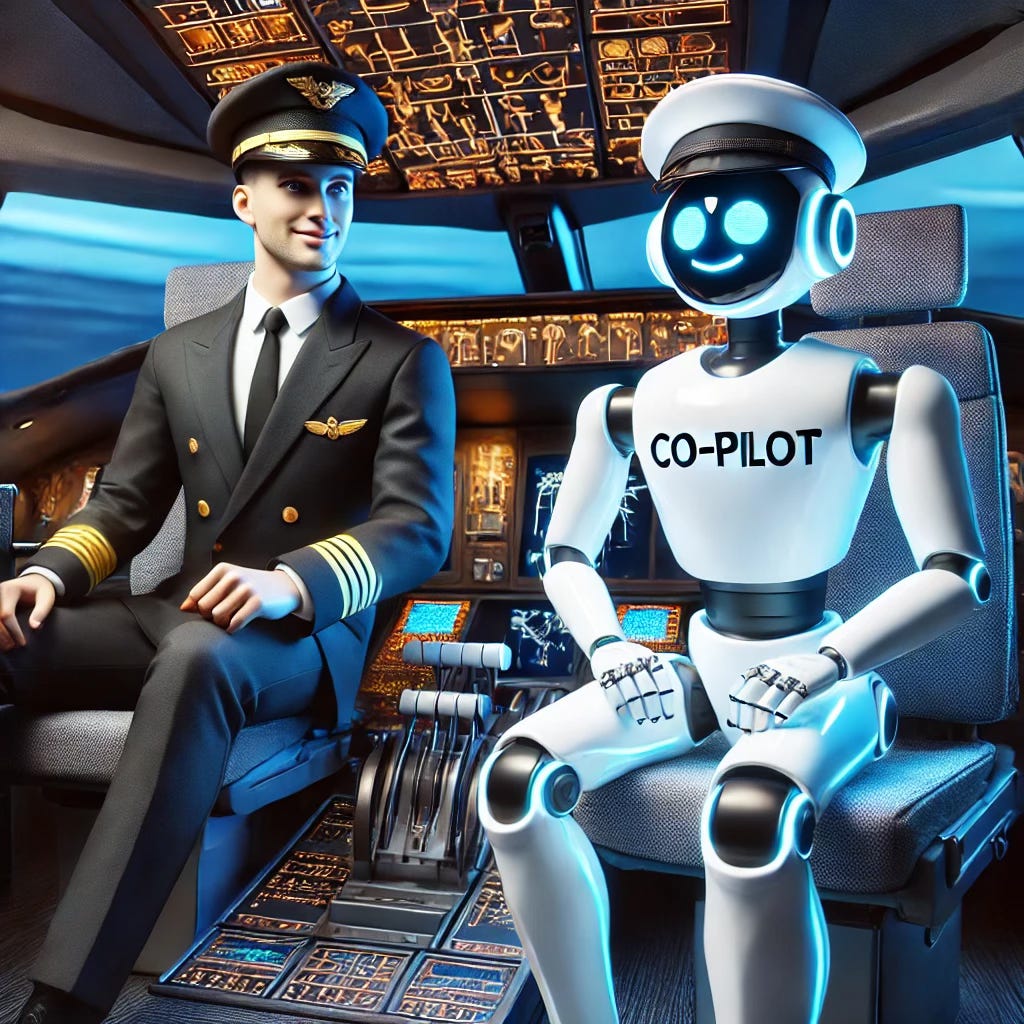
AI readiness needs senior leadership support
This point about AI-driven organisational change being a board-level issue was echoed this week in Larry Dignan’s takeaways from Constellation Research’s recent AI forum.
The board of directors is driving the AI conversation. AI is clearly a boardroom issue, said Betsy Atkins, CEO of Bajacorp. “What boards have figured out is that if they don’t lean in and adopt AI and technology they’re going to be left behind,” said Atkins.
Boards are also being reconstituted for AI. “I see boards shifting in terms of cohorts,” said Atkins, who said enterprises are creating boardrooms that can look at technology as well as new business models to differentiate.
However, the board also wants ROI. Atkins said that enterprises are looking at use cases with quick ROI because boards now realize how expensive AI can be.
He also cited the importance of change management over technology and the imminent need for better orchestration of agents, which are starting to proliferate, albeit still in mostly experimental deployments. Whilst forum participants clearly believed the agentic model will be important, readiness remains an issue.
Elsewhere, Menlo Ventures recently outlined their thesis for agentic AI in a series of posts defining their view of the category and its market:
Fully autonomous agents are defined by four elements that, in combination, ladder up to full agentic capability: reasoning, external memory, execution, and planning.
… At Menlo, we’ve identified three types of agents that vary in their primary use cases and degrees of freedom in controlling the flow of application processes.
At the most constrained end are “decisioning agent” designs, which use language models to traverse predefined decision trees. “Agents on rails” provide one degree more of freedom by equipping agents with a higher-level objective but constraining the solution space with a SOP to follow and a predetermined library of “tools” to choose from. Finally, at the far end of the spectrum lie “general AI agents”—essentially for-loops with little to no data scaffolding on top, that rely entirely on the language model’s reasoning abilities for all planning, reflection, and course correction.
Preparing for an agentic AI future will involve a lot of process and value chain mapping to identify opportunities for autonomous automation. And also a lot of work on internal technology platforms to create the services and service end-points that agents can find and use. And that is before we even start imagining how human oversight will work with lots of real-time agents coordinating tasks and workflows.
Preparing for co-pilot and GenAI usage more broadly is much more of a human skills and ways of working challenge. Companies wanting to discover good use cases for GenAI should be investing in talent, skills development, and piloting AI-supported teams to learn about adoption and potential use cases ahead of a wider roll out.
In a recent HBR article about how a new generation of managers are using GenAI, which also warns of a growing generation gap, the authors shared one simple use case – onboarding – that could help all new staff become more familiar and comfortable with AI:
By leveraging gen AI as a supportive onboarding tutor, new hires can more effectively familiarize themselves with company documents, policies, and schedules, and gain a better understanding of the organization, market and customer needs. This approach helps alleviate the anxiety of appearing incompetent in front of managers and reduces disruption to colleagues. New hires can ask gen AI unlimited questions, 24/7 and in their native language, without worrying that they are asking “stupid” questions or being bothersome to higher-ups.
It would be great to see more organisations sharing their experiences and lessons from AI adoption and AI upskilling, as Dr. Bonnie Cheuk is doing at Astra Zeneca with their Gen AI skills programme.
We would love to hear from more colleagues about their experiences- good or bad – and what lessons they have drawn from early pilots or initiatives.
If you would like to learn more about our hybrid software, services and learning approach to AI implementation, please visit Shiftbase.info