In Don’t Fear the Coming Trough of AI Disillusionment, in January, I wrote:
“I believe what is emerging in enterprise AI has the potential to accelerate the path towards smart organisations that go beyond the limits of the C20th corporation.
But first, we will need to navigate through the trough of disillusionment in the famous Gartner Hype Cycle, and start to value the basics, the long-term marginal gains and the prosaic low-scale automation use cases that will be part of the journey towards AI adoption.”
Well here it is. Buckle up!
The Red Queen Race to AGI
The field of generative AI seems to be entering that trough, and looks set to be battered by a wave of scepticism centred around three key questions:
- Will there ever be a financial return commensurate with the vast amounts of investment required to keep the LLM train chugging away towards AGI?
- Why does linear progress in LLM AI performance require exponentially greater inputs of energy and training content, and is this sustainable?
- Are LLMs a route to real ‘intelligence’, and if not, what other technologies do we need to be working on in combination?
Goldman Sachs recently published a comprehensive and damning report about generative AI that concluded the current massive capex wave will not produce the returns many hope for. Amidst the data and analysis, two interviews in the report are also worth reading:
- MIT Economist Daron Acemoğlu expresses doubt that LLMs are a route to genuine intelligence, and predicts far lower productivity benefits from generative AI than are widely expected.
- Jim Covello, Head of Global Equity Research at Goldman, doubts that LLMs can solve sufficiently complex problems to justify the $1tn he estimates the sector will burn through in the next few years.
If you prefer a more sweary, less dry version of this argument, Ed Zitron’s article on the report is worth a read. He concludes:
“Generative AI is not the future, but a regurgitation of the past, a useful-yet-not-groundbreaking way to quickly generate “new” data from old that costs far too much to make the compute and energy demands worth it.”
“Generative AI is locked in the Red Queen’s Race, burning money to make money in an attempt to prove that they one day will make more money, despite there being no clear path to doing so.”
The Guardian recently covered Google’s 50% rise in emissions caused by its consumption of compute for AI (a problem shared by Microsoft and others), and the debate about the overall impact on energy grids and de-carbonisaton is very much underway. The parallels with the tech sector’s wrong turn towards crypto is rather worrying.
On the content side, Constellation’s Ray Wang is concerned about the ‘garbage in, garbage out’ problem of how LLMs are trained, and how this will be exacerbated by the decline of the open web:
“We will not have enough data to achieve a level of precision end users trust because we are about to enter the dark ages of the internet, where the publicly available information on the internet will be a series of Taylor Swift content, credit card offers, and Nvidia and Apple SEO. Where will the data come from?” said Wang.
Training our emerging AIs on the public output of the internet since the start of the Facebook era seems risky enough in terms of quality, but if you then invite people to further pollute the public web by re-publishing AI-written synthetic content back into places where it can be used as training data, then there is a further risk of a quality reducing feedback loop, and the whole thing starts to smell like a badly managed fish farm.
Way out in the water, see it swimmin’..
But it is the question of the nature of intelligence itself that I think really casts the LLM brute force approach in a negative light.
I “have” a cat. I often marvel at how limited her intelligence appears to be, and yet her abilities and senses are so totally optimised for hunting, eating and managing humans that her ROI on 110g of meat per day is through the roof. I like the stock. She is also psychic and capable of quantum superposition, because she is a cat, obviously.
Nature and evolutionary ecosystems have an incredible ability to eke out every possible gain of function from very limited energy inputs. And most animal intelligence has evolved to make sense of tiny amounts of imperfect, noisy information to survive. I swear my cat’s motion detection vision is about as low-tech as a Tesla’s automatic wipers, but it works through a perfect combination of vision and interpretation at the autonomic level. Elsewhere, despite never having deconstructed a single Reddit thread into a keyword vector space, the Octopus has a form of embodied intelligence and learning ability that we still barely understand.
Intelligence is evolutionary, physical as well as cognitive, driven by goal seeking and needs, and is a super-power of adaptation – a kind of software that can change faster than our hardware.
The human brains that are trying to make AGI happen, each operate at a level of energy consumption estimated to be between 4-6 orders of magnitude lower than Chat-GPT (i.e. Chat-GPT could be consuming the equivalent energy of a million brains). We are currently producing about 140 million new ones every year, at very low cost, and yet we do so little with that existing collective intelligence potential.
At a recent keynote talk about enterprise AI, I suggested people think about generative AI as a social knowledge machine rather than a genuine form of intelligence, although in very narrow, bounded domains it can work very well and appear to be intelligent.
Rather than (literally) boiling the ocean in pursuit of massive, multi-domain AGI, those of us interested in augmenting human abilities and creating more effective organisations to generate more value of all kinds should be starting small and solving real problems. And the technology we already have today can do this, even in the absence of further gains in LLMs.
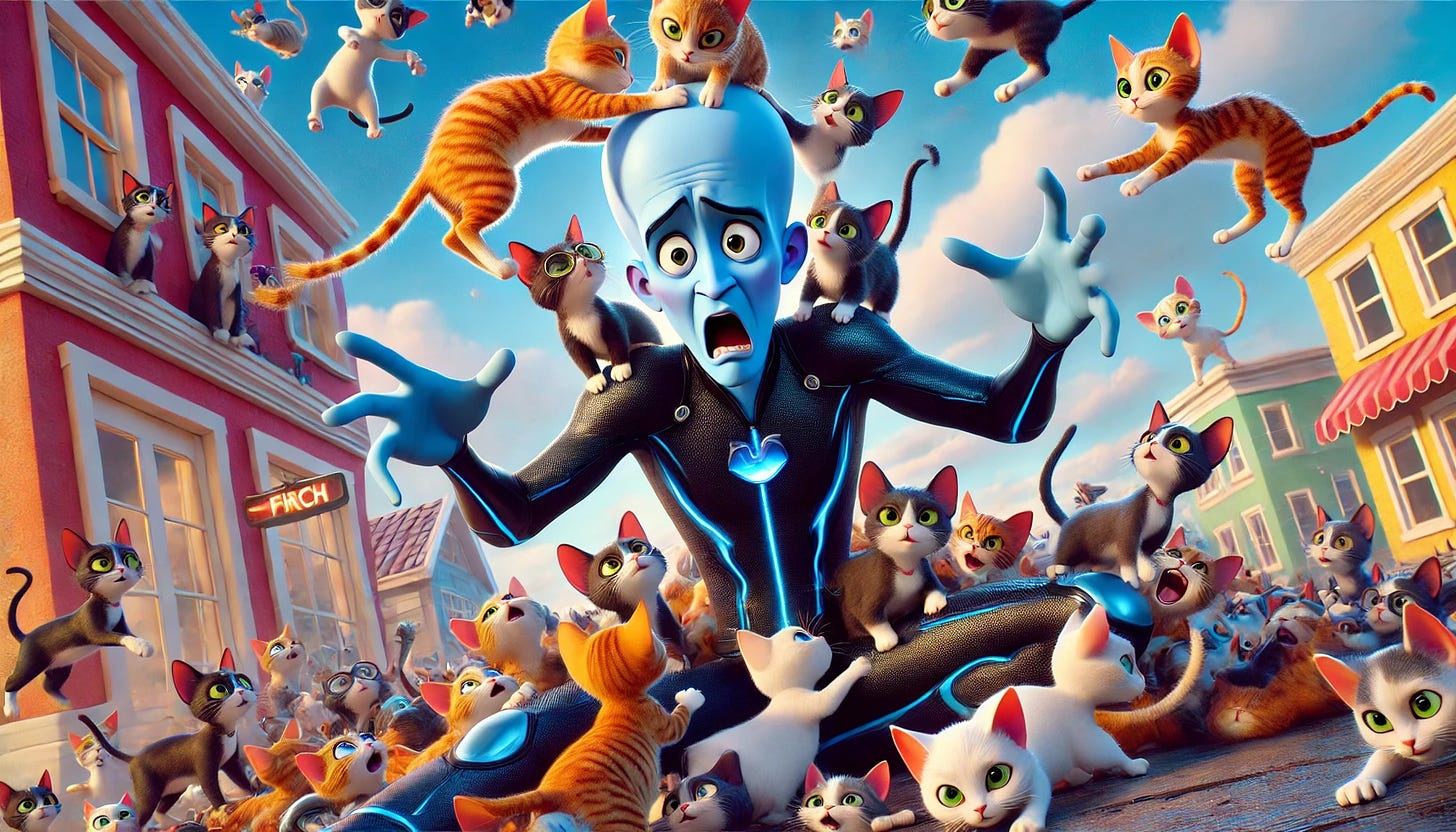
Small Pieces Loosely Joined
The most exciting potential for AI is not replacing people, but connecting them better and augmenting their abilities. In the near term, the most obvious use case for this is to radically improve the ways we do things together: more agile, lower cost, responsive organisations, whether these are companies, government agencies, third sector bodies or just online communities and groups.
As a guiding principle, we should aim to do more with less, reduce costs and energy inputs, and create simpler structures that can evolve and respond to change. Innovation loves constraints. We should not be replicating the LLM model of exponentially greater inputs just to achieve linear improvements in output.
So, it is encouraging to see the rise of small language models that enterprises can start to experiment with today, and adapt to their own comparatively small content stores and data.
But as we argued in relation to centaur teams, the challenge is not to find one AI that can support all use cases, but to create many new service building blocks (we use the term digital business capabilities) that combine AI and human intelligence in a way that be composed, connected and integrated with other services and components. In this approach, the ‘intelligence’ in AI components can be very basic in most cases, but focused on automating tasks that would otherwise be done manually.
We have plenty of prototypes already for some of the basic agent behaviours that will become more widely used. For example, tools like Sana AI show how easy it is to integrate with your calendar, document stores, wiki, etc to create a knowledge agent to support your work, and then connect out to a bigger public GPT to answer more general questions.
If we ignore the noise and the hype around LLMs and AGI, and just start creating these services and building blocks, then the path to value is a lot clearer. Thanks to the over-investment in AI by the big players, there will be lots of cool tools to play with at a low price point as they become more desperate for adoption and proof points. For it is companies, not vendors, who hold the key to progress here: imaginative uses cases and provable benefits.
The outlines of C21st organisations is becoming clearer all the time: modular, composable, platform-based structures that function as an organisational operating system – more like software than the factory model that informed the C20th corporation.
OpenAI co-founder Andrej Karpathy thinks this will be underpinned by a new computing paradigm:
“…with large language models acting like CPUs, using tokens instead of bytes, and having a context window instead of RAM.”
Others, like ourselves and our friends at Boundaryless, think the organisation itself is the brain, not just the compute that powers it, and it will be a new kind of modular, tech-driven organisational OS:
“…corporate teams and units must increasingly be understood as product units. Units should be autonomous and with an end-to-end responsibility to fill one or more user needs and be able to formulate business hypotheses and manage their P&L”
Most likely, these structures will be built – or grown – around small, autonomous teams with the equivalent of APIs and interfaces to connect their work automatically in the background.
But the key attributes a modern organisation needs are to be agile, adaptable, and to learn and evolve routinely driven by feedback, data and real-time results. Starting small and inferring insights from imperfect data, and finding ways to combine and build on simple services is a better route to intelligence than starting with an LLM mega-mind. Ultimately, intelligence is a property of the whole system, which depends on how well adapted it is to the goals and challenges the organisation needs to address.
Small is good. Don’t be held back by trying to predict the future and design a top-to-bottom AI strategy and architecture that will stand the test of time. Just get started with small components and keep finding ways to connect, combine and integrate.
If you would like us to give a talk, run a workshop or help you map your enterprise AI plans and activities, please get in touch any time.