There was a quiet but era-defining shift last week when Google searches in Safari declined for the first time as a result of more people switching to AI tools for finding information. This could have far-reaching consequences both for Google and also for Apple, who have relied on the rent they charge Google to be the default search engine on iPhones.
Whilst increasing AI adoption is the probable cause of this shift, the scourge of ad tech is also to blame. It degrades every user experience it touches, and Google have been guilty of optimising for ad revenue over utility for some time now.
Back in February, Ethan Mollick wrote about the end of search and the beginning of research, and this seems to be coming to pass, with more people using AI services to find information rather than just Googling.
You can start to see how the pieces that the AI labs are building aren’t just fitting together – they’re playing off each other. The Reasoners provide the intellectual horsepower, while the agentic systems provide the ability to act. Right now, we’re in the era of narrow agents like Deep Research, because even our best Reasoners aren’t ready for general-purpose autonomy. But narrow isn’t limiting – these systems are already capable of performing work that once required teams of highly-paid experts or specialized consultancies.
There has rightfully been a lot of focus on the actions that generative AI can perform, but many of its most important and valuable use cases are likely to centre around making the invisible visible or uncovering patterns and signals in real-time that are beyond human view.
Treasure Maps versus shovels
As Sangeet Paul Choudary put it recently in a critique of how enterprise AI is being sold, in Don’t sell shovels, sell treasure maps:
AI lends itself naturally to treasure maps. It has the capacity to uncover hidden relationships across fragmented datasets.
Intelligent solutions can detect small shifts before they escalate and surface weak signals that might otherwise go unnoticed…
Yet, we continue to package AI solutions like traditional productivity software. Just another way to crank out more of the same work. The value of AI is in showing us what we should be doing differently, not in helping us do more of what we already do.
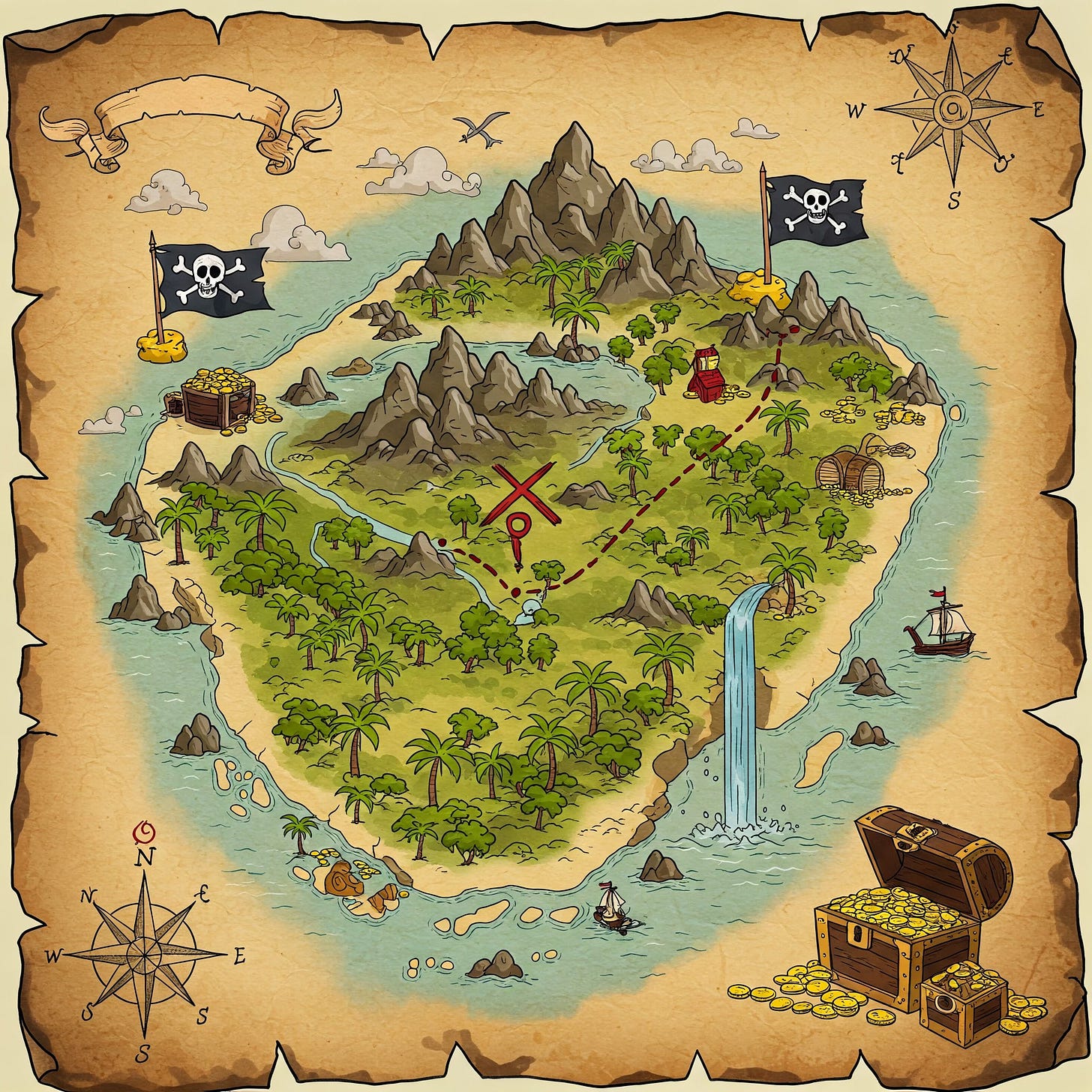
Every new wave of enterprise technology starts with great change potential, but the gravity of sales targets pulls deployment towards shoring up existing ways of working or eking out marginal efficiencies, rather than changing the way work gets done.
No doubt this pattern will be repeated with enterprise AI, but the potential for organisations to radically reduce their operating costs and de-bureaucratise management structures with AI is so great that I am hopeful enough firms will create exemplar enterprise AI operating systems that later adopters will seek to emulate.
Salesforce are starting to talk about the practical, concrete goal of Enterprise General Intelligence rather than the nebulous idea of Artificial General Intelligence (AGI), which is helpful language to focus their customers’ minds on real use cases rather than pipe dreams.
ServiceNow are also pushing hard to become a platform of choice in AI, and predicting the end of big enterprise software systems, to be replaced by an agentic AI operating system. As CEO Bill McDermott put it recently:
The traditional app stack is going to collapse. The number of apps are going to be radically reduced. 20th century systems are going to become core databases.
At the same time, Microsoft are quietly shoring up their incumbent position by evolving CoPilot and extending their own agentic AI models, whilst looking beyond their OpenAI partnership to widen their AI offerings. Rarely the most innovative software firm, they nonetheless enjoy a huge advantage of widespread reliance on M365 and office products, which means that it will make sense for many firms to build on what they already have. And as we have found in our work, the platform is woefully under-exploited by most customers, especially when it comes to the rather fragmented but powerful AI feature set they have been quietly building in the past couple of years. So I wouldn’t count them out.
Making the invisible visible in the enterprise
We focus a lot on the importance of AI readiness, the need for connected data, systems, knowledge, and how a service-oriented architecture is a better starting point for agentic AI than a heavily process-managed structure. But for every quiet CIO hero willing to do the thankless, sometimes invisible work of ensuring enterprise AI readiness, there are probably ten executives who would rather wow a senior leadership meeting with a Gen AI magic show to get budget approval for their proof of concept (which often cannot be scaled or adopted due to … lack of readiness).
However, even where organisations are in the early stages of a transition from top-down process management to laterally-connected teams and platforms, enterprise AI can help accelerate that shift and create the conditions for smarter ways of working. It can do this in two important ways:
- Enhancing visibility and legibility, by mapping teams, value streams, workflows, capacity and capabilities; and,
- Coordinating work information and outputs in real time to ensure passive collaboration and alignment between teams, and optimisation of the value chain.
These affordances can help address the question of the legibility of work structures (mapping) and perhaps eventually obviate the need for ‘manual’ management by doing the water carrying, reporting and coordination of information and work outputs in the background that are necessary for the organisation to function efficiently.
We have had access to proven, non-hierarchical management models for decades, and now we are finally starting to see the drive towards more autonomous work systems becoming a hot topic in HR and organisational development. But many of these examples have relied on visionary leaders (often owners) who are willing to do the hard work of creating, protecting and operating a smarter model. And two of the biggest challenges they have faced are legibility (which hierarchical org charts do pretty well) and visibility of autonomous work.
The most studied case is probably Haier, with its RenDanHeyi model of mutual agreements between autonomous organisational units or micro-enterprises working on a shared platform. But there are various other firms such as Morning Star, Favi and Kyocera, who decades ago decentralised control to empower highly autonomous teams.
Disco is a business unlike any other. Since 2011, it has conducted a radical experiment to operate a blue-chip company on purely free-market principles. Nobody has a boss. Superiors cannot tell juniors what to do. Each day, employees choose whatever tasks they want. They can quit or join a different team at their own volition.
Within this state of perfect freedom, most of their decisions will be guided by Will, as Disco’s internal currency is known. Employees earn Will by doing tasks. They barter and compete at auction with their colleagues for the right to do those tasks. They are fined Will for actions that might cost the company, or compromise their productivity. Their Will balance determines the size of their bonus paid every three months.
It would be fascinating to see how a AI-guided internal marketplace could take this idea further and tweak incentives and prices in the background to optimise performance.
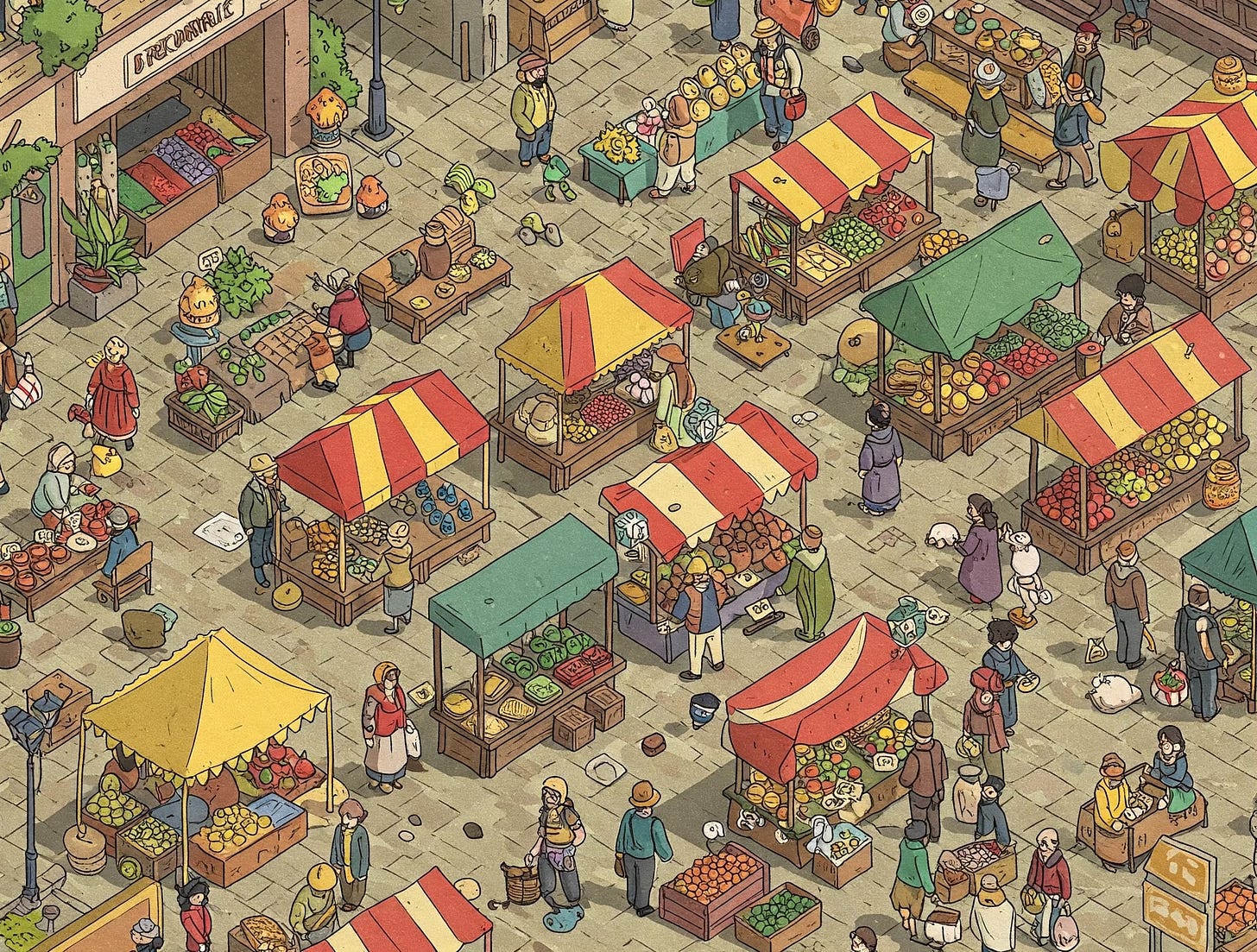
Cybernetic Management Coming into View?
These pioneering organisational models tend to have a few key elements in common that are highly relevant for any organisation trying to improve its work coordination using enterprise AI:
- Modularity: Agent-based orchestration works better in modular organisations.
- Autonomy: When teams can act independently, automation and AI adoption accelerate.
- Incentives and Feedback Loops: Internal currencies, markets or metrics can help AI integrate meaningfully.
- Service orientation: These firms treat internal capabilities as services, critical for AI-driven workflow integration.
Modularity, team autonomy, a focus on services not processes and good incentives and feedback loops all add up to the basics of an evolutionary system that can learn and adapt to change. It is possible to operate such a system when you have strong alignment, a positive work culture and agile leadership and management, but it relies on excellent information and awareness. This is where AI can really change the game.
Organisational operating systems like Haier and Disco are well suited for cybernetic or AI-assisted management systems that aggregate and map information about work agreements, prices, availability, etc. The sheer difficulty and time lag of capturing accurate information from across a complicated system has always been a major practical barrier to the adoption of cybernetic management in the past. But if AI can map, model and synthesise operating information in close to real time, then it is possible to create the visibility and legibility needed to operate more agile and autonomous organisational models.
I can’t wait to see what the next generation of autonomously managed firms are able to achieve with simple AI coordination in the background that makes the system more legible and its functions and capabilities more visible.
In addition to the action modes of enterprise AI, its ability to connect and synthesise operational data is important because it creates the possibility of smarter, more automated and programmable management methods. Minimally, it gives leaders new insights into how the organisation really works by creating a kind of digital twin of the value chain and key functions. But it can do a lot more in terms of automating the flows of data, information and knowledge that people and teams need to stay aligned and maximise their value creation. And this is possible today without any major advances in AI or data technologies, if we do the basics well by surfacing and joining up the data we already generate.