Recent hints by AI researchers that they believe AGI is now within reach have fuelled an increasingly polarised debate about AI harms vs benefits. Ethan Mollick recently wrote up a useful overview of developments that considered where the water is rising (LLM benchmarks, agents, visual GenAI) and what the expected flood of abundant intelligence might look like, and closes by urging us to begin discussing its implications:
even assuming researchers are right about reaching AGI in the next year or two, they are likely overestimating the speed at which humans can adopt and adjust to a technology. Changes to organizations take a long time. Changes to systems of work, life, and education, are slower still. And technologies need to find specific uses that matter in the world, which is itself a slow process. We could have AGI right now and most people wouldn’t notice (indeed, some observers have suggested that has already happened, arguing that the latest AI models like Claude 3.5 are effectively AGI).
Elsewhere, I see more critiques and push-backs coming from technological, political, economic and cultural points of view on the risks of AI. We should not dismiss them, but nor should we be paralysed by fear of the future.
But at the same time, we are also only just seeing the most basic enterprise use cases coming to fruition, and they do not seem particularly risky.
Small is Beautiful in Bounded Domains
We have previously predicted that areas such as professional and financial services that have the advantage of a tightly bounded system of language would be among the early AI adopters; and this is starting to happen. Lawyer Monthly recently asked whether AI in legal would make lawyers obsolete (spoiler alert: not for the foreseeable future), and argued for more focus on how people and AI can collaborate to achieve the best results – similar to what we are calling centaur teaming.
Today, John Gapper in the Financial Times covered three Legal AI startups in the UK who are making an impact on the way companies handle basic legal matters such as contract drafting. Whilst prestigious law firms are still addicted to the hourly rate, which disincentives greater efficiency, their clients are discovering that they can do a lot more legal work quicker and cheaper in-house, partly thanks to GenAI:
Companies can upload thousands of existing contracts into AI models, which analyse the clauses and terms they tend to use. The technology can use that knowledge to help to draft new ones, showing where changes are needed. Luminance’s platform highlights in red and amber clauses that require human attention and can suggest reworkings to ensure they comply.
This is rather like having an experienced lawyer by the side of an employee who is preparing a contract: they can easily and cheaply call on a large database of knowledge. The ability to search past contracts efficiently has other benefits. Companies can, for example, easily find which clients they must inform of a cyber attack instead of hiring a law firm to carry out a review.
In a similar vein, Business Insider report that Goldman Sachs claims AI is now able to do 95% of the work on creating IPO prospectuses, which would previously have involved junior staff grinding away at document assembly.
From Document Drafting to Better Risk Management
The scope for this kind of use case has already been demonstrated in procurement and other repetitive document-heavy functions. But the potential use cases are far wider.
For example, in regulation and compliance, why rely purely on manual compliance and post-facto review, when we have the data to map events in close to real time? In the FT today, Dan Davies critiques the bureaucratic rear-view mirror approach of a European Banking Authority paper on using AI to analyse bank failures, and hopes for a better future where we can use already existing datasets to predict or avoid such failures.
If you want to dig deeper into how AI could help organisations build cybernetic control systems that are more effective than manual command and control systems, then David Dagan’s roundup in the Hypertext journal – How to give bureaucracy back its brains – is worth a read, and is also partly inspired by Dan Davies’ book The Unaccountability Machine:
It is tempting to cast bureaucracy as merely an “arm” of a government (or corporate management), controlled fully by elected officials (or board members) who act as the “brain” and are directly accountable to the citizenry (or shareholders). But that simple, anthropomorphized metaphor simply isn’t fit for purpose in a modern economy. Instead, we might turn to a military metaphor, in which elected officials are the generals and the bureaucracy forms the platoons. Those platoons understand the mission and the rules of engagement and are given the autonomy to execute within those parameters. To do that, bureaucracy cannot just be a limb. It needs a brain of its own.
Each of these use cases for AI carries some risk, and this needs to be assessed. But what the legal AI startups understand is that generic LLMs are probably not the way to go here, but rather (as one firm, Luminance, put it) Legal Pre-Trained Transformers could be more effective and reduce the risk of hallucination that general-purpose LLMs still suffer from. Plus, of course, the highest value form of Reinforcement Learning from Human Feedback (RLHF) is real professionals offering their insight and review into AI-produced documents and policies.
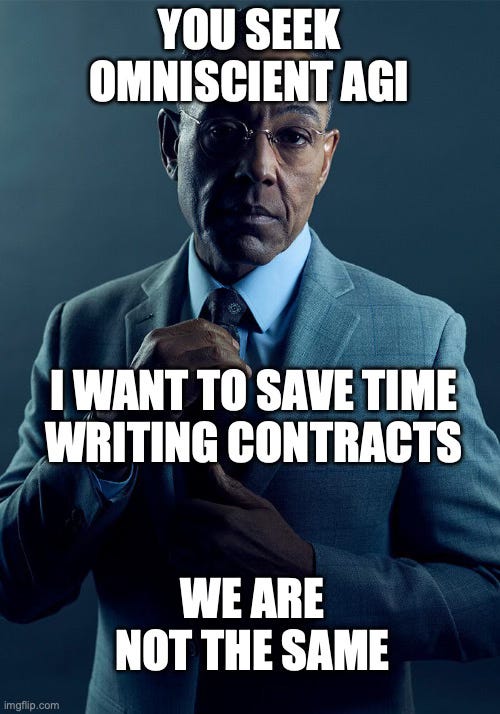
Enterprise and Consumer Tech are Not the Same
In AI, as in previous waves of social computing, we need to remember that enterprise and consumer fields are quite different in terms of pricing, addressable market, risks and rewards. The concerns people rightly have about the risks of big-picture general-purpose AI are not to be ignored, but they don’t apply to enterprise AI in quite the same way.
Yesterday’s US inauguration will be remembered for many reasons, but from a technology perspective, it encapsulated the frightening power of social media to change popular culture and politics in rather worrying ways. And the sight of big tech bosses lining up to pay tribute to the new emperor, whilst the beaten down ‘mainstream media’ tied itself in knots to politely cover proceedings without saying what they really think was … quite something to behold. As Henry Farrell insightfully pointed out, social media has not just contributed to a proliferation of disinformation, it has also degraded democratic publics, which is altogether much harder to recover from.
In the brief period between its genesis and the rise of Facebook, social media looked like it could connect and educate people, create new forms of cooperation, and overcome the limited but dominant lens of mainstream media in shaping perceptions. But incentives are everything, and none of these benefits had incentives as powerful as the basic click-bait model of monetising social media through advertising. We are still trying to quantify the social harms that rage bait and engagement forming have caused, but the overall report card is not exactly positive.
As social technology pioneers, we witnessed both the early excitement and the subsequent disappointment around the direction that social media took. Blogs hosted respectful debates between people who disagreed with each other. We shared our life photos on Flickr without worrying that they might be harvested and used against us. Micro-blogging was mostly about keeping in touch with real world friends and acquaintances, rather than trying to become a professional influencer. And trolling was seen as bad behaviour, rather than a route to fame and influence, as we have seen with the rise of dystopian figures such as Curtis Yarvin in the United States.
Our focus, then as now, was on using these social technologies to improve the way people collaborate and work together, and although this was also trend-driven, it had a very simple fitness function: if companies found it useful to invest in over time, and if stakeholders found it improved their experience of work, then that was validation enough. This new field was broadly positive in its impact, both for bosses and workers, and unlike consumer social media, it didn’t create huge external harms beyond a touch of chat fatigue.
Some Lessons from Social Media
There are a few lessons that emerge from looking at Enterprise vs consumer social media, which could help us navigate the benefits and potential harms of AI and set a course for enterprise AI that avoids some of the wider risks of consumer AI.
First, the scale and bounding of social groups and knowledge domains really matters.
If you want a productive discussion between professional colleagues on a complex topic, then a smaller, more intimate setting will work better than a wide open forum that creates incentives for grandstanding and attention farming. Similarly, if you want to train an LLM or AI on a very specialised area of professional knowledge, a smaller model trained on high quality sources might be better than one trained on the whole of the internet.
Related, is the question of who takes responsibility for local community standards and rules.
Communities of practice have existed within organisations and professional disciplines for a long time. They typically each have their own culture and rules, and it is better to connect or federate them on this basis rather than try to enforce global lowest-common-denominator rules across them. Especially when, as we have seen recently, big tech firms can flip a switch to change the defaults of acceptable behaviour on a whim to curry political favour.
Third, skin in the game and the right incentives are important.
When people are connected by reputation, employment contracts, company guidelines, or even just physical proximity, they tend to behave in a more measured way than random internet trolls. When we first started popularising wikis as a feature of enterprise knowledge systems, managers used to worry about how to deal with defacing content or crazy behaviour and we would respond by asking when they last saw an employee arrive at work and spray paint graffiti in the corridors. It doesn’t tend to happen when people share a measure of common purpose, reputation and basic rules. We need to think carefully about the incentives that surround the use of AI inside organisations.
And finally, it is all about the use cases.
Consumer AI, like crypto, risks incentivising anonymous exploits to garner attention and make money. Anyone can make magic app wrappers around generic LLMs and find an audience, at least for a time. But enterprise AI is all about actual utility and its outcomes are testable, at least in the sense that companies believe it is contributing to the bottom line or a more productive future top line. One criticism of enterprise social computing is that too often it was sold as being worthy or the-right-thing-to-do rather than based on concrete, provable use cases.
World Building, not Renting
Enterprise AI use cases are typically either too small for big tech to care about or too difficult to achieve quickly (smart factory automation, office automation, knowledge agents, etc). But if they succeed, then the total value created (and bureaucratic cost savings) will be enormous, albeit diffused widely across markets. There will be dominant players in software, such as Microsoft, ServiceNow, Salesforce, but there may not be a Facebook, Twitter or TikTok that is able to capture as much value as they have in the consumer space, and that is undeniably a good thing for businesses.
Organisations have an opportunity to define and build their own ‘worlds’ with their own rules and culture, and they can adapt tools, systems and content to become an ‘owned’ part of their own organisational operating system, rather than just accept whatever big tech AI offers as part of their solutions.
To be fair to social media, some niches and micro-climates still exist where people try to be nice to each other, objective or useful, but on the whole it feels like a rather stupid and toxic culture is the norm in public spaces because the scale is too big and the intimacy too fake and/or transactional. It would be nice to think we can avoid making the same mistake with consumer AI, but just to be safe, it is a good idea to treat enterprise AI as a distinctly separate application area.
This also means that many of the fears and risks people have about AI can be mitigated within an organisation, whether it is concerns about copyright, replacement of workers, AI-enabled surveillance or AI ‘going rogue’. And in future, the richness and capabilities of these internal worlds could become a key differentiator for companies that want to attract the best talent, just as foosball tables, snack bars, inclusive policies and working from home were a decade or so ago.